Generative art has long been one of the most prominent use cases for machine learning (MI), but only recently has the area acquired widespread significance. The development has been primarily driven by computational advancements and an emerging class of techniques that enable programs to acquire knowledge without requiring a large number of classified data sets, which are extremely scarce and very expensive to create. In addition to the fact that the divergence that exists between the generative NFT art community and AI research has been narrowing in recent years, many of the new generative art techniques have not yet been broadly adopted by renowned artists, as it takes time to explore the latest approaches.
The Drivers for Generative Art
Even many of the earliest AI innovators, who viewed generative AI as a comparatively hidden subset of machine learning, have been surprised by its rapid rise. The remarkable advancements in generative AI can be attributed to three primary factors:
Multimodal AI: In the past five years, there has been an explosion of AI techniques that are capable of working across multiple domains, including language, pictures, videos, and voice. This has allowed for the development of models that create videos or images from natural language.
Pretrained language models: The recent development of multimodal AI was supported by significant advancements in language models using techniques such as GPT-3. This has made it possible to use language as an initial mechanism to generate artistic results such as images, audio, and videos. In this new phase of generative AI, language has played a crucial role by lowering the obstacle that allows individuals who want to communicate with generative AI models to do so.
Diffusion models: The majority of photo-realistic art created by AI techniques today depends on a technique known as diffusion models. Prior to the introduction of diffusion models, the generative AI space had been ruled by techniques such as generative adversarial networks (GAN) and variational auto-encoders (VAE), which have difficulty expanding and generate outputs that lack variety. Diffusion models circumvent these limitations by corrupting the input data pictures until they are completely corrupted by noise and then reconstructing them. If a model can reassemble an image from something that is, in theory, noise, then it should be able to do so from virtually any domain, including other domains such as language.
The Processes Fueling Generative Art in NFTs
Text to image: Text-to-image (TTI) transformation is the most prevalent application of generative AI in the NFT community. Some AI models created in the TTI space are literally transcending into popular culture. Google has played around with generative NFT art, testing various techniques such as Imagen, which is based on diffusing models, and Parti, which is based on a technique known as autoregressive modeling. Meta has also fostered the generative art community with tools such as Make-A-Scene. AI startups are also making inroads in the TTI space.
Text-to-video: Text-to-video (TTV) is a more difficult element of generative art, but one in which significant progress is being made. Meta and Google have recently released TTV models, including Make-A-Video and Imagen Video, that can generate high-frame-fidelity video segments based on natural language. Media is one of the most researched areas of generative art, so we should anticipate that the majority of the generated image models have video equivalents. Videos are currently less prominent in the NFT space than visuals, but this is expected to change as more generative artists adopt TTV models. Video is one aspect that distinguishes digital art from conventional art.
Image-to-image: Image generation based on text sources feels almost natural but has constraints when it involves recording elements such as positions between various objects, orientation, and even extremely particular characteristics of the surrounding environment. The most effective way to share this information is through drawings or other visuals. The majority of the most well-established generative art practices center on the creation of images from other images. Unexpectedly, several popular NFT collections of generative NFT art are founded on variants of image-to-image methods.
Music generation: Generating music automatically is yet another prevalent application of generative AI that has garnered prominence in recent years. OpenAI has been at the cutting edge of this transformation with models that can produce music in a variety of styles and genres.
Conclusion
Within the course of the development of technology, there have been a number of cases in which somewhat separate trends have influenced one another to achieve enormous market share. Generative NFT art and AI are beginning to show a similar dynamic. The two developments have succeeded in bringing a complicated technological market to the forefront of popular culture. In the absence of NFTs, it would be virtually impossible to implement digital ownership and distribution models. Likewise, generative AI will undoubtedly become one of the most significant drivers of NFT creation.
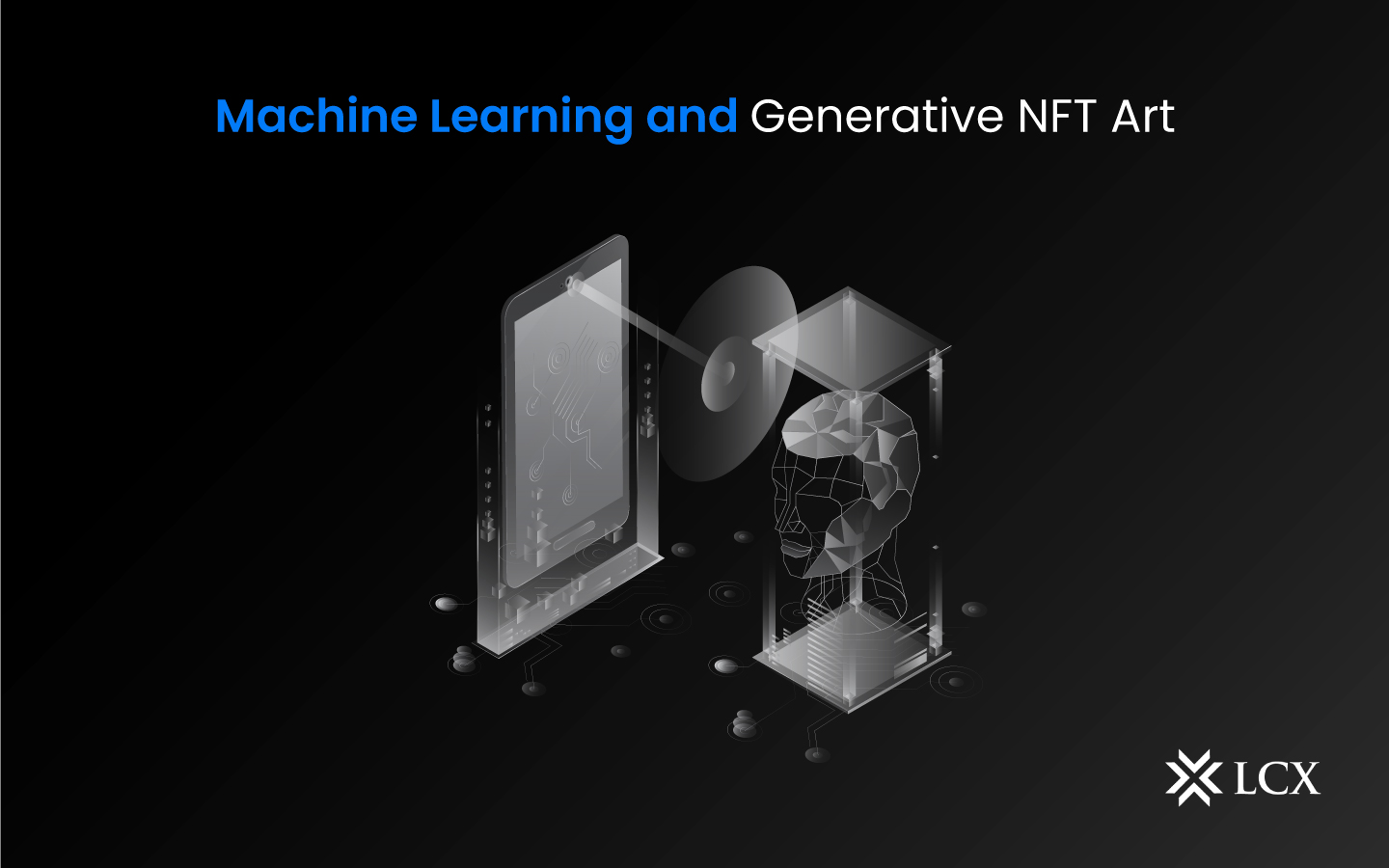